Rethinking our love of metrics
- Vijaymohan Chandrahasan
- Feb 17
- 5 min read
Updated: Feb 25
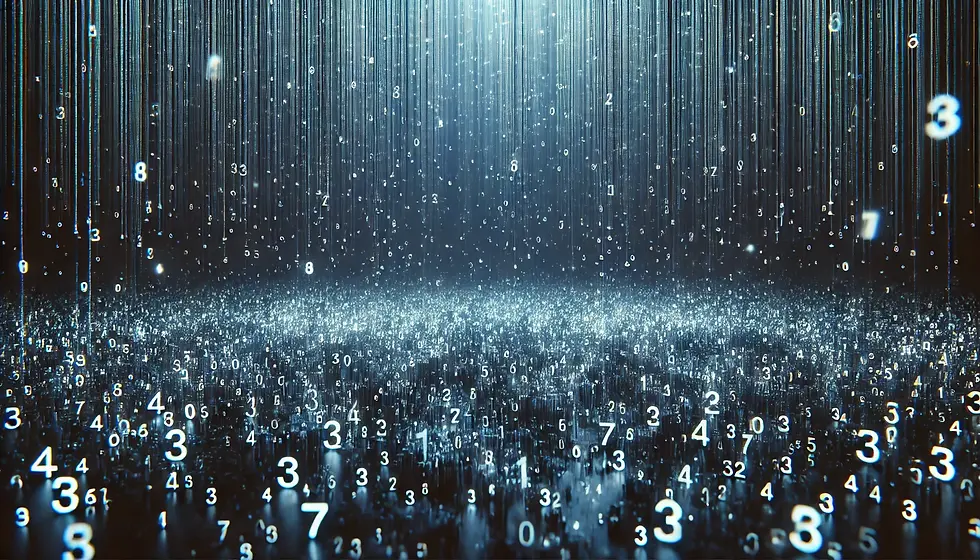
I can’t count how many times I’ve heard someone declare, with a hint of smugness, “Numbers don’t lie.” There’s this unshakeable conviction among some teams - often self-described as “numbers people” - that data is the ultimate arbiter of truth.
Yes, numbers are powerful. They provide a certain clarity in a world that can feel unpredictable. But what I’ve come to realise is that while numbers themselves might not lie, our interpretation of them absolutely can.
The lure of tidy metrics
Not long ago, I saw a team present an incredibly high click-through rate for one of their email campaigns. On the surface, it looked like they’d discovered a perfect formula - everyone in the room was celebrating. But when I began correlating those numbers with end-to-end data over a few months, a more complex story emerged.
It became clear that, despite the familiar practice of using end-to-end analytics, the real insight lay in looking across a longer period. Patterns began to form: catchy subject lines and compelling CTAs enticed users, but the actual offering behind the click sometimes fell short of expectations. Over time, we realised that if users feel misled - or if the next step isn’t as valuable as promised - those brilliant-looking click-through metrics don’t necessarily translate into genuine engagement. It’s not that the numbers were wrong; they were simply incomplete, capturing only the initial burst of interest rather than the whole experience and trust.
This wasn’t about blaming the team or dismissing the value of their work. The click-through data was accurate within its scope. But it was only part of a broader picture - one that included true user satisfaction (or lack thereof) once they arrived on the landing page, and the journey thereafter. In fact, subtle areas of disappointment had built up over time, eventually eroding trust even when subsequent campaigns were more genuine. What initially appeared to be a “win” ultimately revealed deeper issues with aligning user expectations and actual product or service value.
When data presentations mislead
It’s not just about the numbers themselves; it’s also about how they’re shown. One high-profile case involved Apple’s M1 Ultra launch. On the surface, Apple’s data suggested its new chip was vastly outperforming a competing Nvidia GPU with far less power consumption. But a closer look revealed that the chart’s scale was truncated, giving the impression of a bigger performance gap than there actually was. The numbers Apple provided weren’t necessarily “incorrect”; they were framed in a way that magnified the M1 Ultra’s relative performance.
The danger here is that people often absorb the visual cue more than the fine print. Even with solid data, the way you visualise it can steer interpretation in a specific direction - sometimes to the point of being misleading. I’ve trained myself to question any especially dramatic chart: where does the axis start, and what timeframe or context is missing? What comparisons are (or aren’t) being made?
Correlation is not causation
I’ve also seen teams jump to conclusions about what caused a specific data spike or dip. They’ll note that usage soared immediately after a new feature launch and treat it as proof that the feature was an instant success. Sometimes that’s true, but it might also coincide with a robust marketing push, a holiday season, or a competitor’s outage. Without controlling for other factors, we can’t assume it was our change alone driving the needle.
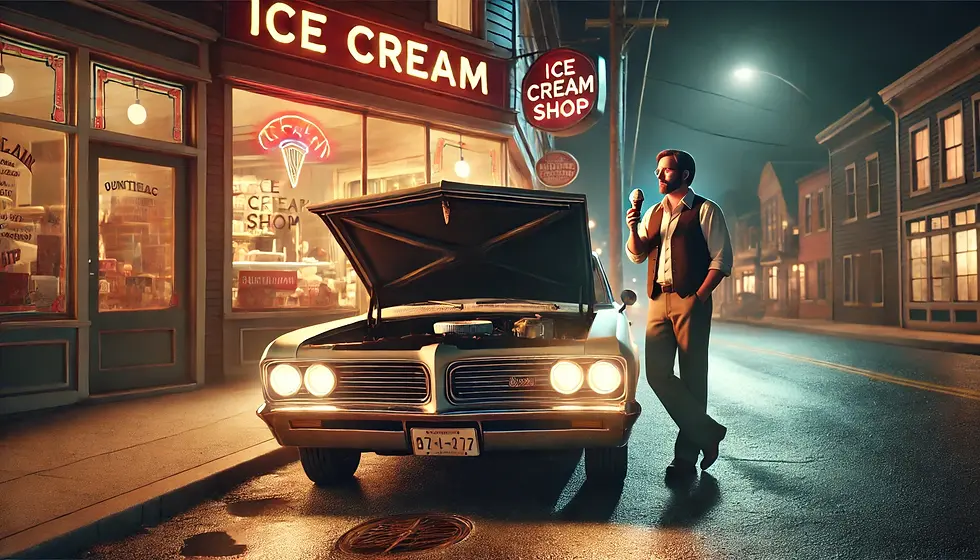
A favourite illustration (often told as an urban legend) involves a complaint to the Pontiac Division of General Motors: a man claimed his car wouldn’t start when he bought vanilla ice cream, yet worked fine with other flavours. Initially, it sounds absurd to connect vanilla ice cream to engine troubles. But upon investigation, an engineer discovered that vanilla was located near the entrance of the store, so purchasing it took less time. Because the trip was so short, the engine didn’t cool properly and experienced vapor lock when restarted. The issue wasn’t the flavour of the ice cream; it was the timing of the purchase.
This story, despite its potentially mythical origins, underscores a real principle: if you see two events happening at the same time, it doesn’t necessarily mean one is causing the other.
Looking at the entire journey
If there’s one concept I can’t stress enough, it’s the importance of viewing the full journey. Metrics might spike in one place, but if people abandon the site or product soon afterward, that initial data point doesn’t mean much in the grand scheme.
Collaborating across different teams - marketing, user research, product development, and customer support - provides a richer perspective. One group might highlight impressive click-through rates, while another uncovers a surge in support tickets because users found the landing page design confusing. When we combine all these vantage points, it becomes much easier to address issues holistically. You begin to see whether you’re genuinely meeting user needs or just throwing up attention-grabbing headlines that fail to deliver deeper value.
Also, it’s critical to consider how metrics evolve over time. A layout tweak might cause an initial dip in conversions because users need to adapt. But if, a few weeks or months down the line, data shows conversions rising beyond previous levels, the dip may have been worth it. By contrast, a short-lived spike can come crashing down if the feature doesn’t genuinely solve user problems.
Balancing quantitative and qualitative approaches
Numbers excel at showing what is happening. But if you want to understand why it’s happening, you often need to speak directly with users or observe their behaviour through usability tests. I can recall instances where an A/B test suggested that moving a button increased clicks. At first, it seemed like a clear win. Yet, when we interviewed users, many said they found the new layout disorienting. The extra clicks weren’t necessarily indicative of better engagement - it turned out some users were clicking multiple times just trying to figure out how things worked.
This is where a balanced approach to data becomes vital. Quantitative analytics can identify interesting trends or anomalies; qualitative research can shed light on what drives them. If your metrics show a sudden spike in sign-ups, a few user interviews might reveal whether it’s due to the feature’s merits or because of a temporary promotion that won’t sustain long-term growth.
Long-term thinking and user trust
One of the toughest lessons I’ve learned is that beneficial changes don’t always pay off right away. Improving accessibility or including clearer unsubscribe options, for example, might not show up as a big win in this quarter’s metrics. However, in the long run, these user-centric decisions can foster loyalty, reduce churn, and build a reputation for integrity.
Of course, it’s hard to justify investments without immediate data to back them up. But the fact remains:
When users feel respected and see that your product delivers on its promises, they’re more likely to stick around.
Conversely, if they feel tricked by inflated claims or manipulative tactics - even once - it can sour their perception for months afterward, and fixing that kind of trust deficit is an uphill battle.
Conclusion
Data is a powerful guide, but it’s not a silver bullet. A single eye-catching metric might point us in the right direction - or it might send us chasing illusions. The key is context: recognising how data is presented, acknowledging that correlation doesn’t necessarily imply causation, and looking holistically at the entire user experience.
Whether you’re celebrating an impressive click-through rate or a sharp rise in user engagement, it pays to ask: “What happens after the click? What do these numbers mean for trust and satisfaction over the long haul?” By blending hard data with qualitative insights and by engaging multiple teams - from marketing to product to research... you stand a far better chance of designing solutions that genuinely resonate with users.
I believe the best outcomes happen when we treat metrics not as the final word, but as signposts guiding deeper exploration. Rather than focusing on vanity wins, we can aim for sustainable improvements that genuinely benefit people and in the process, earn the kind of loyalty no flashy chart can guarantee.
Comments